Vital and Sensor Data Processing
The ongoing digital transformation is currently leading to major changes in many areas of society. In the healthcare sector, for example, it is making itself felt through the increased use of telemedicine and e-health and, as a result, the rapidly growing use of mobile systems / wearables for recording and processing vital data. The health data available in real time and the possibility of analysing trend data enable a wide range of applications from permanent patient monitoring and accident prognoses to extended fitness tracking. There are two main challenges for the development of such mobile systems: Firstly, there are limitations to the usability of the sensor data due to a lack of robustness of the signal under motion/physical activity. Secondly, many mobile systems only have limited resources available that can be used to correct and further process the data. In recent years, methods from the field of machine learning have increasingly been used alongside classic signal processing techniques to reduce artefacts and further process vital data.
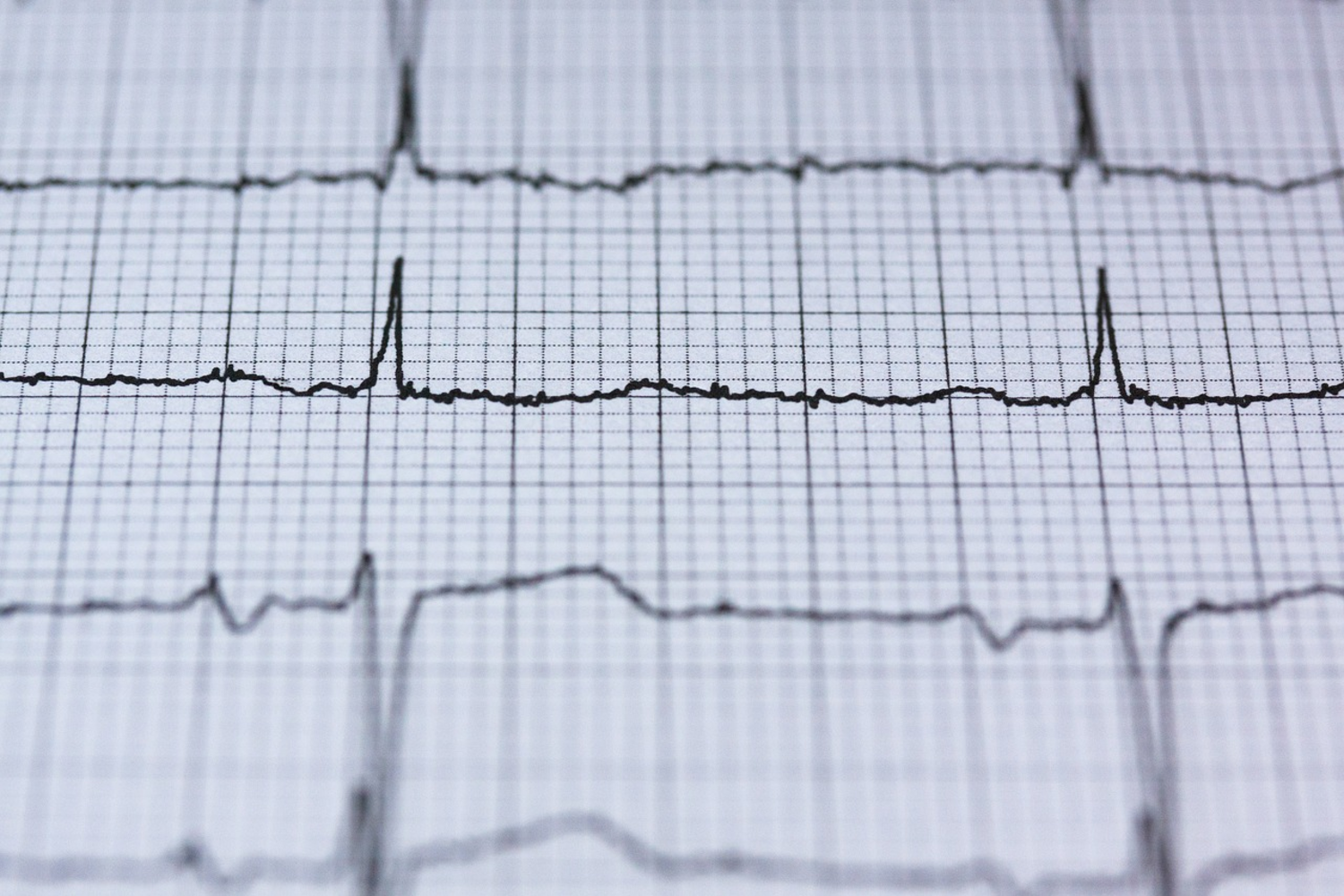
Deep PPG
Contact: Prof. Dr Andreas Breidenassel
Duration: 2021-2023 (3 years)
Funding: Struktur Impuls
Partner: OSRAM Opto Semiconductors GmbH, Regensburg
Associated partner: Prof. Dr O. Amft (FAU Erlangen)
Research associate: Maximilian Reiser
Cardiovascular diseases are the most common cause of death in Germany. Medical wearables that measure vital parameters such as blood pressure, heart rate and oxygen levels in the blood in real time could help to recognise these diseases at an early stage and treat them preventively. The small, portable mini-computers in the form of fitness wristbands or smartwatches are already very popular in everyday life or during sport. They are now also being used more and more frequently in medicine. The problem here, however, is that the mobile systems are not always free of errors. For example, most wearables measure vital parameters using the so-called PPG method (photoplethysmography). This can lead to signal interference if, for example, the sensors slip during movement. However, medicine depends on reliable measurements. This is precisely where the new "Deep PPG" research project at Landshut University of Applied Sciences, headed by Prof Dr Andreas Breidenassel, comes in. Its aim is to reduce the susceptibility of the PPG signal to interference and thus enable more precise measurements of wearables in medical applications. The company OSRAM Opto Semiconductors is involved in the project. The project is funded by the Bavarian State Ministry of Science and the Arts(more).
M. Reiser, A. Breidenassel, O. Amft, Simulation framework for reflective PPG signal analysis depending on sensor placement and wavelength, 2022 IEEE 18th International Conference on Wearable and Implantable Body Sensor Networks(Link)
Edge AI
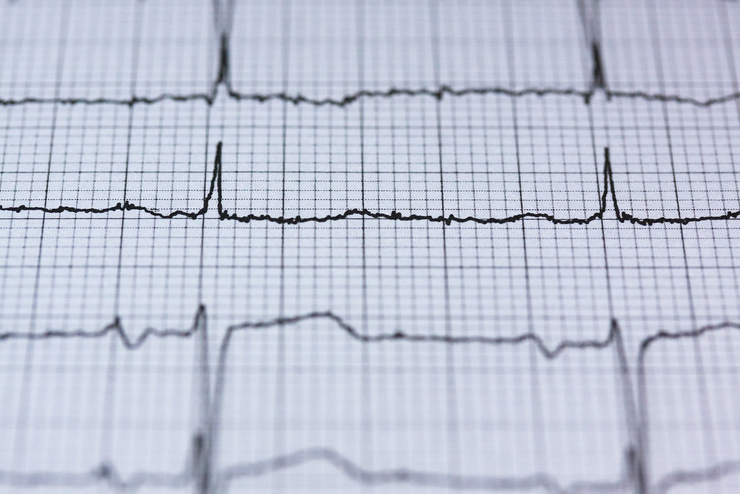
Classification of ECG data using deep learning on an Nvidia Jetson Nano system
Project work in the Master's programme in Electrical Engineering: Leo Hurzlmeier, Christiane Huber
Diseases of the cardiovascular system (CVD) are the most common cause of death worldwide. These include cardiac arrhythmias, which can be diagnosed using an ECG. A precise diagnosis is necessary in order to choose the right treatment for the right type of arrhythmia. This proves to be time-consuming and tedious and should be reduced through the use of computer-aided diagnostic programmes. In the 2019 winter semester, a project group successfully classified ECG data using deep learning. Three different clinical pictures were categorised with a reliability of 98.06%. The influence of noise was also investigated and the system was implemented on the Nvidia Jetson Nano.
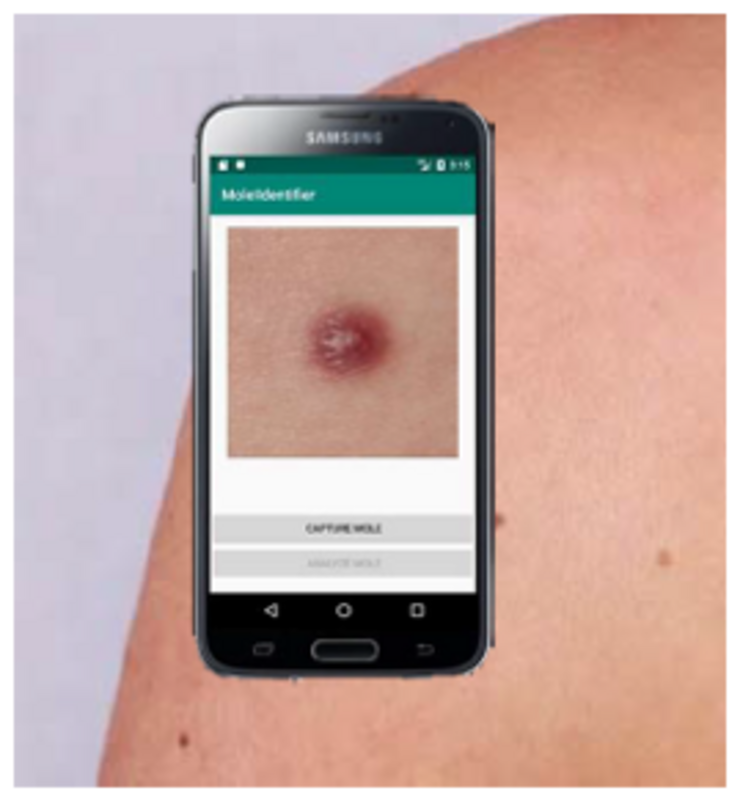
Mole Identifier - Mobile classification of skin lesions
Project work in the Master's programme in Electrical Engineering: Maximilian Reiser, Gabriel Horkovics-Kovats
The risk of developing skin cancer has risen from 0.17% to between 1.00% and 1.33% since the 1960s. A decisive factor in reducing the mortality rate of 14.11% (as of 2010) is early detection before metastasis. In one project, a method for classifying skin lesions was developed using depp learning and a mobile phone camera. Different trained models can be selected in the application. The user can then take a picture of the skin lesion to be classified with the mobile phone camera and have it evaluated. The segmented skin lesions were classified with an accuracy of 85.11%, the trained network was ported to an Android smartphone and controlled with an app.
Embedded Systems
EMG Biofeedback - System
Project work in the Master's programme in Electrical Engineering: Michael Hartl, Michael Gröber, Matthias Laber, Lisa-Maria Kirchner (project work)
Michael Gröber, Paul Wingert
Project partner: Gerald Gradl(Texas Instruments)
A mobile biofeedback system was developed as part of a project. With the help of the system, patients can, for example, focus their awareness on the paralysed areas in order to train them in a targeted manner. It can also be used as a warning system, e.g. for people in a tense posture (office work at the computer) or as a training system for athletes. A signalling pathway for evaluating muscle potential was designed, then simulated and measured using a test set-up.
Conception and prototypical realisation of a tonometer
Project work in the Master's programme in Electrical Engineering: Witte, Tesik, Eilers
The intraocular pressure is decisive for the survival of the organ. If the intraocular pressure is permanently elevated, this can lead to damage to the optic nerve later on. The project group has built a mobile prototype of a tonometer. In addition to the control board and coil system, a power management system was developed. A PIC24 was used as the microcontroller and the housing was manufactured from polyactide using 3D printing (fused deposition modelling - FDM).