Medical Imaging and Image Processing
Medical care goes hand in hand with analysing and using a very large and constantly growing amount of medical data. Imaging diagnostics, for example, is one of the two main pillars in the detection and diagnosis of diseases. The task of radiologists is initially to create medical images using MRI, CT and co. The images are then either examined for abnormalities (detection) or the presenting clinical picture is characterised (diagnosis), depending on the issue at hand. This diagnostic process is time-consuming and tedious in view of the large volumes of data involved. Very time-consuming and complex image processing procedures are also often necessary for the planning and management of minimally invasive interventions in therapy.
The research field of medical image processing has therefore been dominated for several years by machine learning topics, especially with the help of deep neural networks (deep learning).
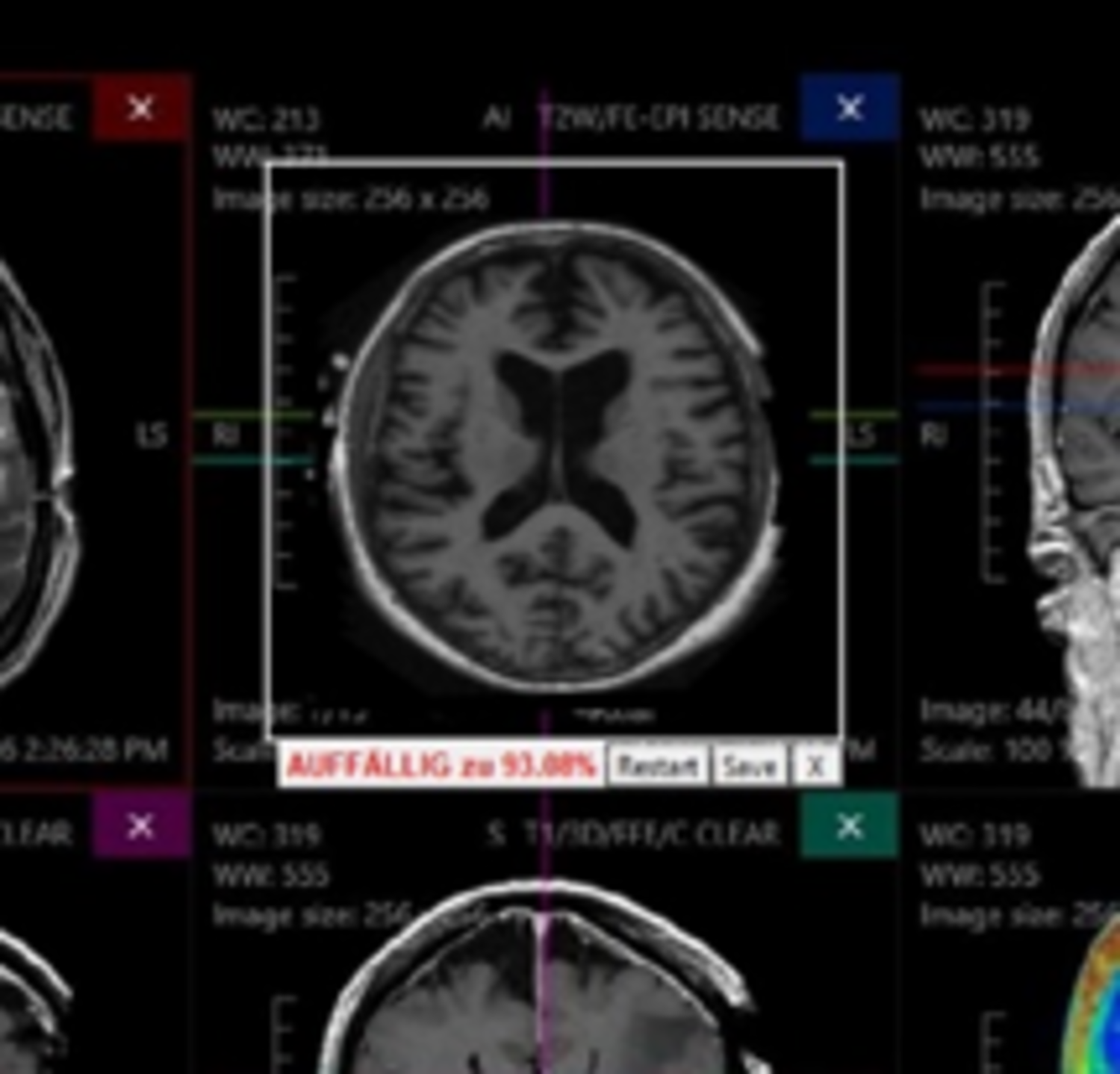
NeuroTEST
Contact: Prof Dr Stefanie Remmele
Duration: 2021-2023 (2 years)
Funding: ZIM
Partner: deepc GmbH, Munich
Associated partners: LAKUMED Krankenhaus Landshut-Achdorf, Prof Dr Tobias Schäffter (PTB Berlin, TU Berlin)
Research assistant: Christiane Posselt
Machine learning methods, in particular using deep neural networks, are celebrating ever new successes in the analysis and classification of medical images. It is clear that in many applications, the algorithms are already achieving at least human-like decision-making accuracy. However, it is still unclear how networks trained on a specific data situation will behave in a different data situation. For example, when imaging hardware or imaging parameters differ from radiology to radiology.
In the BMWi-funded ZIM project NeuroTEST, the Medical Technology research group (project lead Prof Remmele) is developing methods for the systematic validation of neural networks (use case: segmentation of MS lesions) together with the Munich start-up deepc. To this end, methods of statistical experimental design are being researched, whereby AI algorithms are systematically tested on data from different recording protocols. The project at HAW focuses on the simulation and synthesis of this data in order to be able to provide arbitrary data domains for the stress tests.
AI supported and AR guided interventions
Projects and funding:
- AIARLiver: Internal (2021 - 2024)
- INMOTION: STMWK Bavaria (2024 - 2027)
- AIARConcepts: Internal (2024 - 2027)
Clinical partners: Agatharied Hospital, Bayreuth Clinical Centre, LAKUMED Hospital Landshut-Achdorf
Industrial partners: Cariad SE, Corscience GmbH, Cosinuss GmbH, Infineon AG, mediCAD Hectec GmbH
Professors:
Prof. Dr Stefanie Remmele (Image Processing & Synthesis)
Prof. Dr Christopher Auer (Mixed Reality)
Prof. Dr Eduard Kromer (AI Algorithms)
Prof. Dr Norbert Babel (3D Printing)
Prof. Dr Aida Anetsberger (Medical support)
Research assistants: Serouj Khajarian, Michael Schwimmbeck
This research focus area investigates technologies to support surgeons using mixed reality technologies that allow target and risk structures of an intervention to be superimposed on the patient. The focus is on AI-supported algorithms, for example for tracking and the precise visualisation of models on the patient (registration) and concepts for implementation in medical applications.
The Free State of Bavaria is funding the "INMOTION - Innovative Motion Tracking Technologies in Medical Applications" initiative as part of the 7th funding round of the programme to promote applied research and development at HAWs and THs with a funding amount of €714,977. The university is supporting the project by funding a qualification position to set up the research group (AIARConcepts).
Deep learning for segmentation and object detection
Contact: Prof Dr Eduard Kromer
Partners: Fraunhofer Institute for Process Engineering and Packaging IVV (Dr Thilo Bauer, Satnam Singh), precipoint GmbH (Ludwig Wildner)
Bachelor theses: Katharina Bauer (BMT), Lena Kinzel (BMT) in 2021
In various projects, also in collaboration with industrial partners, the university is investigating and developing deep learning models for regression, semantic segmentation and object detection for processing two- and three-dimensional medical image data. The focus here is on the traceability of the results, the type and detail of the output and the generalisability of the models (also to non-medical data).
Visualisation methods for the validation of deep convolutional networks
Contact: Prof Dr Stefanie Remmele
Bachelor's thesis: Jakob Dexl (2018)
In the summer semester of 2018, various visualisation approaches were implemented for the CNNs. These are intended to make the network's decision-making process comprehensible and thus help, among other things, to identify weak points in the algorithms and optimise the methods as well as to draw attention to the detected anomalies in the long term. The work was honoured with the VDE Prize in the Science category in 2019.